OVERALL GOAL: Develop a Digital Twin and Machine Learning algorithms for addressing key food safety challenges associated with introduction and spread of pathogens in food facilities and enable guided decontamination for food contact surfaces to reduce risk of cross-contamination.
GENERAL GOALS: The proposed approaches are divided into both fundamental and applied approaches.
Fundamental Approach #1) Computational framework for real time computation using digital twin models: The overall goal is to develop a computational framework for digital twin models that can be run in real time with the physical system. The examples of these frameworks include parallel compute functionality in agent-based models to characterize spread of pathogens in a food facility based on interaction among people, food, equipment surfaces and processing operations.
Fundamental Approach #2) Integration of validated digital twin model for AI enabled prediction of risks of spread of pathogens and decontamination effectiveness: This project will provide foundation for integrating multifactorial output from digital twin models to train machine learning models.
Success in developing this framework will enable industrial deployment of these models and provide rapid response associated with characterizing the risk of spreading of pathogens and sanitation and decontamination.
Project Part I
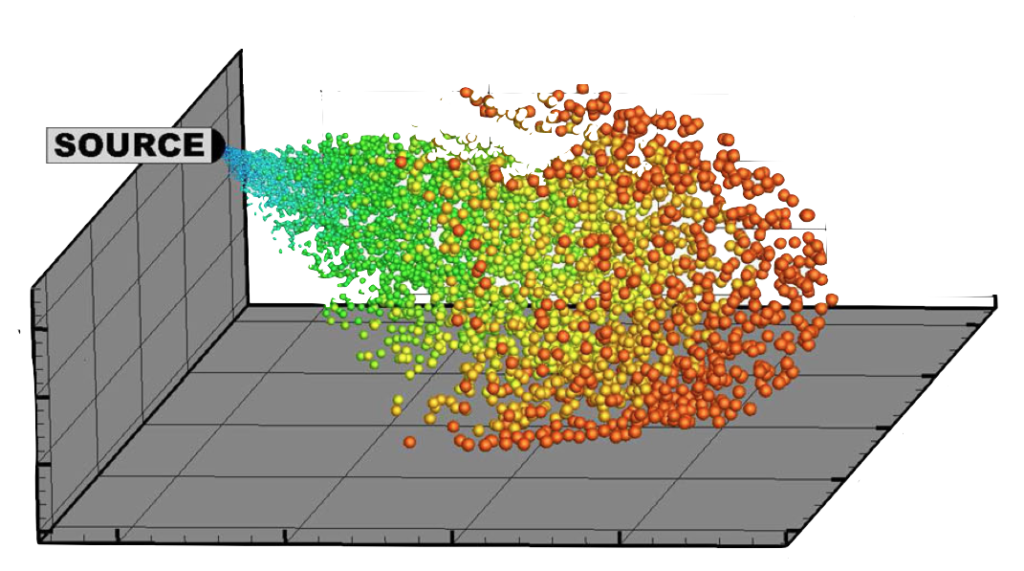
Cough simulation. (Zohdi, https://msol.berkeley.edu/)
PROJECT PART I: We focus on two food safety scenarios:
(a) The spread of viral pathogens (Covid-19) in a simulated work environment based on particle dynamics under diverse air flow and ventilation conditions
(b) The spread of Listeria in a processing facility based on interactions among people, equipment and food products.
For each of these scenarios, the team will develop digital twin models to predict the biophysical dynamics of pathogens, validate these digital models using experimental data sets using surrogates under simulating industry conditions or field data and use validated models to train machine learning based predictions for the spread of pathogens in food facilities.
GENERAL GOALS- PROJECT PART I:
Applied Approach #1) Particle transport models of viral dispersion and spread: Digital twin models simulating the spread of viral pathogens through droplets will be modeled. The influence of ventilation conditions and worker spacing will also be simulated in modeling the risk of infection. In addition, the transfer coefficient and dispersion of viruses through food contact surfaces to humans and produces will also be simulated.
Applied Approach #2) Agent based models for pathogen spread in a food facility: The increase in readily available computational power raises the possibility that direct agent-based modeling can play a key role in the analysis of epidemiological population dynamics. Specifically, one objective of this work is to develop a robust agent-based computational framework to investigate the emergent structure of Susceptible-Infected-Removed/Recovered (SIR)-type populations and variants thereof, on industrial scales. The work will illustrate the construction of computational frameworks with direct, non-phenomenological, input data.
Project Part II
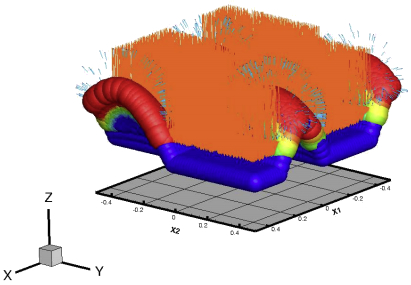
UV-c pulse applied to a surface. (Zohdi, https://msol.berkeley.edu/)
PROJECT PART II: We plan to evaluate guided decontamination of the food contact surfaces using light based antimicrobial technologies including UV light.
The proposed research plan will build on the digital twin models to predict the spread of pathogens as well as experimental approaches to predict the localization of biofilms on food contact surfaces. The outputs of the models and the experimental data will be used for developing machine learning models to guide deposition of light energy and predict inactivation of viruses and biofilms.
GENERAL GOALS- PROJECT PART II:
Applied Approach #1) AI enabled detection of biofilms using imaging/spectroscopic approaches: We propose to develop experimental data sets using imaging/spectroscopy measurements to enable detection of single and multispecies biofilms on diverse food contact surfaces. Applied machine learning models such as gradient boosting decision tree will be developed based on experimental spectroscopic data set for detecting the presence of biofilm on diverse surfaces. Machine learning models will also be developed to identify differences in biological composition of the biofilms as these composition differences may impact the inactivation efficiency. This involves non-invasive and rapid detection of biofilms on diverse food contact surfaces.
Applied Approach #2) Digital twin models for the inactivation of viral particles and biofilms: We propose to develop digital twin models integrating physical models of light propagation and absorbance in complex biofilm architecture or food contact surface topography and biological models of bacterial and viral l inactivation based on UV-c and photochemical activated approaches. These digital twin models will train AI models to deliver adequate dosimetry for the inactivation of viral particles on diverse surfaces and surface attached biofilms. AI based predictions will be validated using experimental measurements. This involves a digital model to predict inactivation of biofilms and training of AI guided inactivation.
Project Team
Collaboration
The projects are jointly conducted with partners through the AIFS Network:
https://aifs.ucdavis.edu/