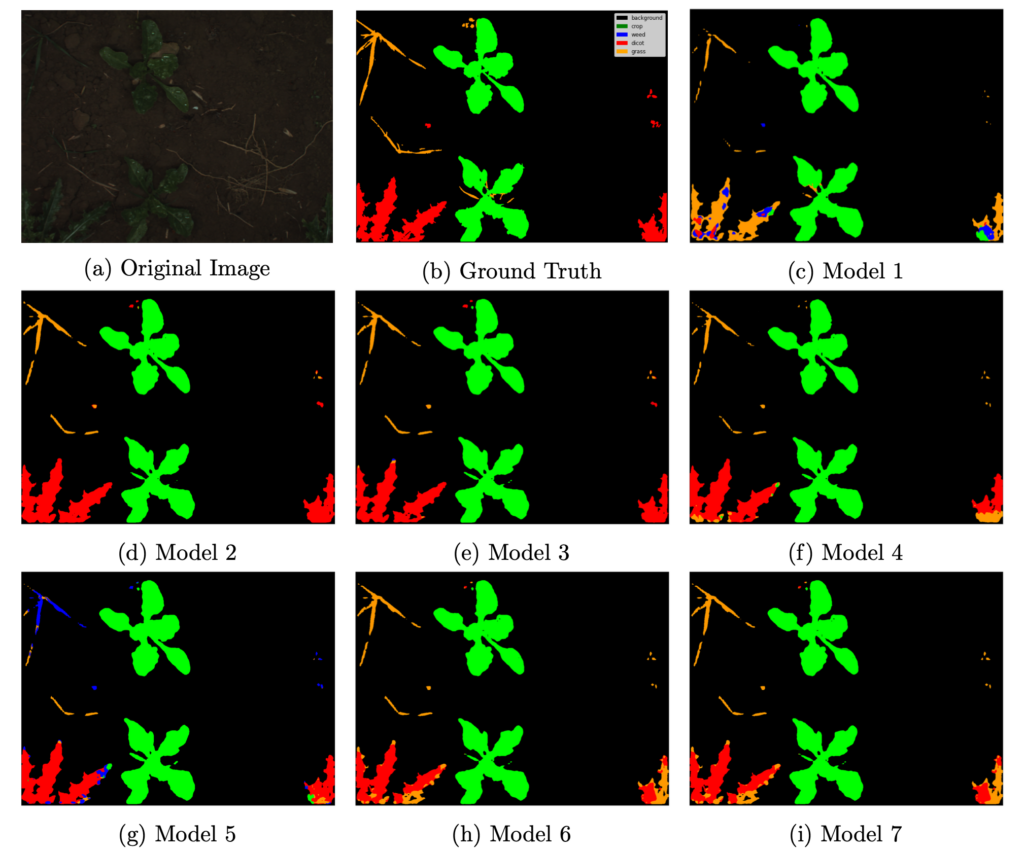
PROJECT DESCRIPTION
A robust and viable framework for uncertainty quantification in food systems is Performance Based Food Engineering (PBFE). PBFE methodology can be broken down into four main processes, which are: (1) environmental hazard analysis, (2) crop growth analysis, (3) crop yield analysis, and (4) loss/downtime analysis. A fifth process can involve a secondary collateral analysis. More details can be found here.
To capture and quantify the uncertainty in different crop growth stages, one promising tool is Deep Learning (DL). DL-based approaches have been gradually utilized to tackle computer vision problems in the agricultural field over recent years, and one emerging, yet challenging task, is semantic segmentation of crop images. Our team has developed DL-based models for field weed detection and real-time monitoring of crop growth conditions. The deep architecture that has been used for our purpose is the Transformer, which was proposed in 2017 and has become the de facto model over the last four years in the field of Natural Language Processing (NLP). The transformer architecture has also been applied to the computer vision domain and outcompetes Convolutional Neural Networks (CNN’s) in some vision tasks. Specifically, an efficient yet powerful transformer-based semantic segmentation framework, SegFormer, has been executed on field crop images to predict the masks of crops and weeds; Fractional Green Canopy Cover (FGCC) values, as a crop growth measure, have been evaluated based on the outputs of the framework. By comparison, our model outperforms a widely used online tool for measuring FGCC, Canopeo. One typical challenge experienced for applying our developed model is the lack of consistency between the images in terms of several important parameters, such as the distance between the camera and the plant, the camera angle, etc. However, this problem can be easily tackled for the proposed indoor farming systems. With cameras mounted at particular locations in the pods (e.g., pointing at some plants), images taken in a fixed interval (e.g., one image per hour) will achieve consistency and can be processed by our model for quantifying, monitoring and evaluating plant growth conditions.
RESEARCHERS
PI: Khalid Mosalam, GSR: Fan Hu
This work is supported by the USDA/NSF AI Institute for Next Generation Food Systems (AIFS) through the AFRI Competitive Grant no. 2020-67021-32855/project accession no. 1024262 from the USDA National Institute of Food and Agriculture.